Artificial intelligence’s fundamental subfield is machine learning. It enables computers to enter a self-learning mode without the need for explicit programming. When given new data, these computers learn, evolve, adapt, and develop on their own.
Long many machine learning techniques have been around for a while, and the ability to apply difficult mathematical computations to enormous amounts of data automatically – over and over, faster and faster – is a recent development.
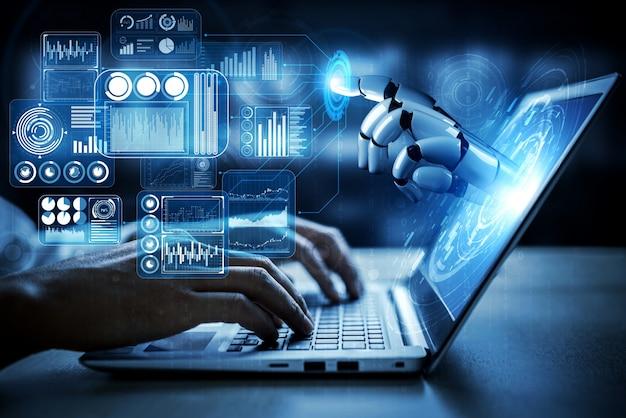
To get you started, here are a few well-known examples of machine learning applications:
- The much-hyped Google self-driving car? Machine learning at its most fundamental level
- Do online recommendation services such as Amazon and Netflix exist? Applications of machine learning in everyday life.
- What are your customers saying about you on Twitter? Machine learning and the creation of linguistic rules are being combined.
- Is it possible to detect fraud? In today’s climate, this is one of the more obvious and critical applications.
What is the Importance of Machine Learning for a Data Scientist?
In the not-too-distant future, process automation will supplant the majority of human labor in manufacturing. To match human abilities, devices must be intelligent, and Machine Learning is at the heart of AI.
Data Scientists must understand Machine Learning in order to make accurate forecasts and estimates. This can assist machines in making better decisions and taking wiser actions in real-time without the need for human intervention.
Machine learning is revolutionizing data mining and interpretation. Traditional statistical procedures have been replaced by more accurate automatic sets of generic algorithms. As a result, Machine Learning skills are essential for Data Scientists.
4 Must-Have Skills Required to Become a Machine Learning Expert
To become an expert in Machine Learning, every Data Scientist requires the four skills listed below.
- Computer fundamentals require a deep understanding and competence. Only a few examples are computer organization, system architecture and tiers, and application software.
- Because Data Scientists’ jobs need a lot of estimation, a solid understanding of probability is critical. Statistics analysis is another area on which they should focus.
- Data modeling is used to investigate different data objects and how they interact with one another.
- It is necessary to have programming skills and a thorough comprehension of programming languages such as Python and R. A desire to learn new database languages, such as NoSQL, that aren’t traditional SQL and Oracle.
Who’s using it?
Most firms that deal with large amounts of data recognize the usefulness of machine learning technologies. By gleaning insights from this data – often in real-time – organizations can perform more effectively or gain a competitive advantage.
- Financial Services
Machine learning is used by banks and other financial institutions for two main purposes: finding meaningful insights into data and preventing fraud. The data can be utilized to identify investment opportunities or to provide trading advice to investors. Data mining can also be used to discover clients with high-risk profiles, or cyber monitoring can be used to spot fraud warning indications.
- Government
Because they have various sources of data that may be mined for insights, government organizations such as public safety and utilities have a special need for machine learning. Sensor data, for example, can be utilized to find ways to save money and increase efficiency. Machine learning can help with both the identification and prevention of fraud.
- Health care
Because of the introduction of wearable gadgets and sensors that can use data to analyze a patient’s health in real-time, machine learning is a fast-growing trend in the healthcare business. Medical experts can use the technology to evaluate data and identify trends or red flags that could lead to more accurate diagnoses and treatment.
- Retail
On websites that propose goods you might like based on previous purchases, machine learning is utilized to analyze your purchasing history. Machine learning is used by retailers to collect, evaluate, and apply data to personalize a shopping experience, launch a marketing campaign, price optimization, inventory supply planning, and consumer insights.
- Oil and Gas
Finding new sources of energy. Mineral analysis in the earth. Sensor failure in refineries can be predicted. Oil distribution is being streamlined to improve efficiency and lower costs. In this field, the number of machine learning applications is huge – and growing.
- Transportation
To increase income, the transportation industry relies on making routes more efficient and detecting future problems, therefore analyzing data to find patterns and trends is crucial. Machine learning’s data analysis and modeling capabilities are critical for delivery firms, public transportation, and other transportation enterprises.
Conclusion
Data analysis is essential for identifying patterns and trends. By 2022, it is expected that the global demand for Data Scientists will have increased by 48%. Finance, insurance, professional services, and IT will account for 59% of the demand for Data Science and Analytics jobs.
Machine Learning will be one of the greatest options for analyzing large amounts of data in the future. As a result, in order to boost their productivity, Data Scientists must obtain a deep understanding of Machine Learning.